ARTIFICIAL INTELLIGENCE (AI), INTELLIGENT EXPLORATION
5 Things You Should Embrace if You Want to Be Successful with AI in 2023
.png)
Written by Virtualitics
Jan 3, 2023 9:00:26 AM
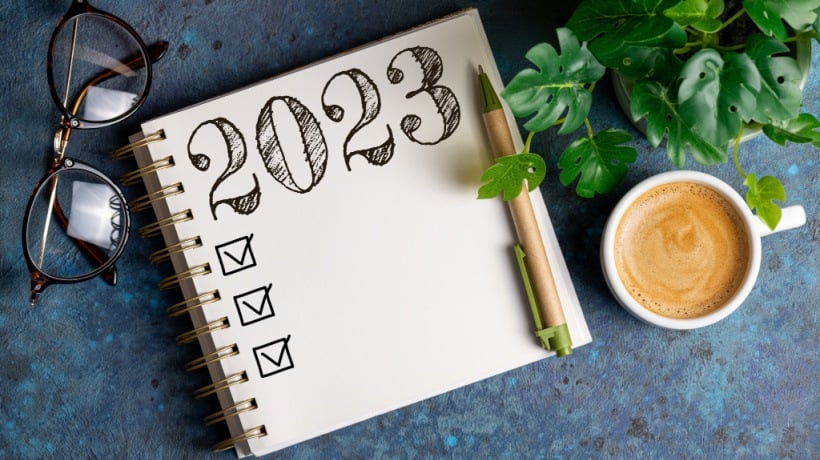
It’s a new year and with that comes a clean slate and the best of intentions to get things right! If you have “Build a Successful AI Program” at the top of your 2023 resolution list then here are 5 things that you need to embrace.
1. Rediscover the Lost Art of Data Exploration–This Time with AI
Many AI use cases will fail from a lack of appropriate data exploration early on in the process. The old tools that are in use for basic analytics, while still useful, are not getting the job done for exploring the vast data that are relevant for AI use cases. And considering that AI is intended to automate decision-making and action-taking at scale, making sure that you’re pursuing the right use case and using the right data sets is critical.
In 2023, don’t try to validate hypotheses using basic BI to justify your next AI use case. Instead, leverage AI algorithms to explore business challenges with an open mind. This will bring more options, reduce bias, and ensure that you’re following up on high-impact possibilities. To learn more, read What is Intelligent Exploration?
2. Finally(!) Start Using Modern Data Visualizations
Fully interactive multidimensional visualizations are the next standard in advanced data analytics. They are game-changers for both front-end exploration of data and for illustrating the findings and implications of your AI algorithms. Analysts and data scientists that try to show complex data relationships in traditional business intelligence tool visualizations will fail to communicate them clearly, they won’t get informed buy-in, and they could actually lead organizations down the wrong path.
AI-generated true 3D visualizations make the relationships between multiple data points consumable. Instead of a rabbit hole involving the comparison of myriad pie charts, histograms, and scatter plots, true 3D illustrates the connections concisely. We’re not talking about forced-perspective 3D, either. We’re talking about visualizations that can be manipulated like an object in space, rotated by the observer, and pulled in to zero in on a particular point. We’re talking about native 3D visualizations. To create better AI, you need more data; to find the insights in more data, you need more robust visualizations.
3. All Things Network Graph
Gartner analyst Rita Sallam said the research and advisory firm forecasts that 80% of data and analytics innovations will be made using graph technology by 2025 and the market is expected to grow by 28%. But most heads of Analytics have only a passing familiarity with network graphs and how they can be used at the Enterprise level. It’s no surprise, really. The tech stack and methodology have been complex, meaning that most data professionals just don’t have any experience.
So for 2023 your goal should be to learn more about what network graphs can do, because they’re more accessible than ever before with AI-powered Network Extractors. Now teams are able to create persona profiles of high-churn customers, spot the weak spots in a supply chain, and analyze any highly connected datasets. Read more in our Beginner's Guide to Network Analysis.
4. Find Beauty in Simplicity
We were all blown away by the capabilities of GPT-3 (an advanced AI-powered search, conversation, text completion app) and perhaps dream of unveiling something that vast and ambitious ourselves. But for 2023, let’s take a moment to appreciate the beauty of a simple solution. Just because AI is the mission doesn’t mean that AI is always the answer. With just over half of AI projects getting to production, it’s pretty clear that we could be doing a better job vetting the use case list.
The ability to spot the best, most effective path forward is where robust exploration in the preliminary project phases really pays off. Once you’ve surfaced your potential use cases, look at possible solutions from all angles, and include business stakeholders who will have a different perspective. When considering feasibility, don’t forget to count technical costs, and change management costs, alongside everything else. And if you come to the conclusion that the best solution is not an AI model? That’s a win, proof of the team’s value, and shows you have a thoughtful assessment process. Read more about Finding the Right AI Use Case.
5. Responsible AI Means Ownership of the Model
Much is said about responsible AI–AI that is transparent in its predictions and is thoughtfully developed to account for bias and downstream impact. But nothing can be truly responsible without accountability. Frustratingly, once deployed, AI models seem to become orphaned, with no one taking ownership. If something goes wrong in production, where does the buck stop? And if no one is responsible once the model is applied, who feels the responsibility during the development process to make sure that the model itself is responsible?
Data and Analytics leaders should consider clearly defining roles and responsibilities for all AI projects for the business. These responsibilities should include providing the business with clear information about what data is being used, how it is being used, and the impact the model had during testing in a format that business stakeholders can understand. It is the responsibility of the business stakeholders to consume this information and ensure that they understand it. A plan for remediation should be in place should the model drift or not perform as expected and someone should be named to monitor the model. Furthermore, users should be empowered to understand the model, and understand when they should challenge the model’s results.
Are you ready to make Intelligent Exploration, 3D visualizations, and AI part of your 2023 game plan? Contact us for a personalized demonstration.