Risk and Reward: The Struggle of Data Sampling
Written by Amanda Derrick
Mar 8, 2023 9:18:39 AM
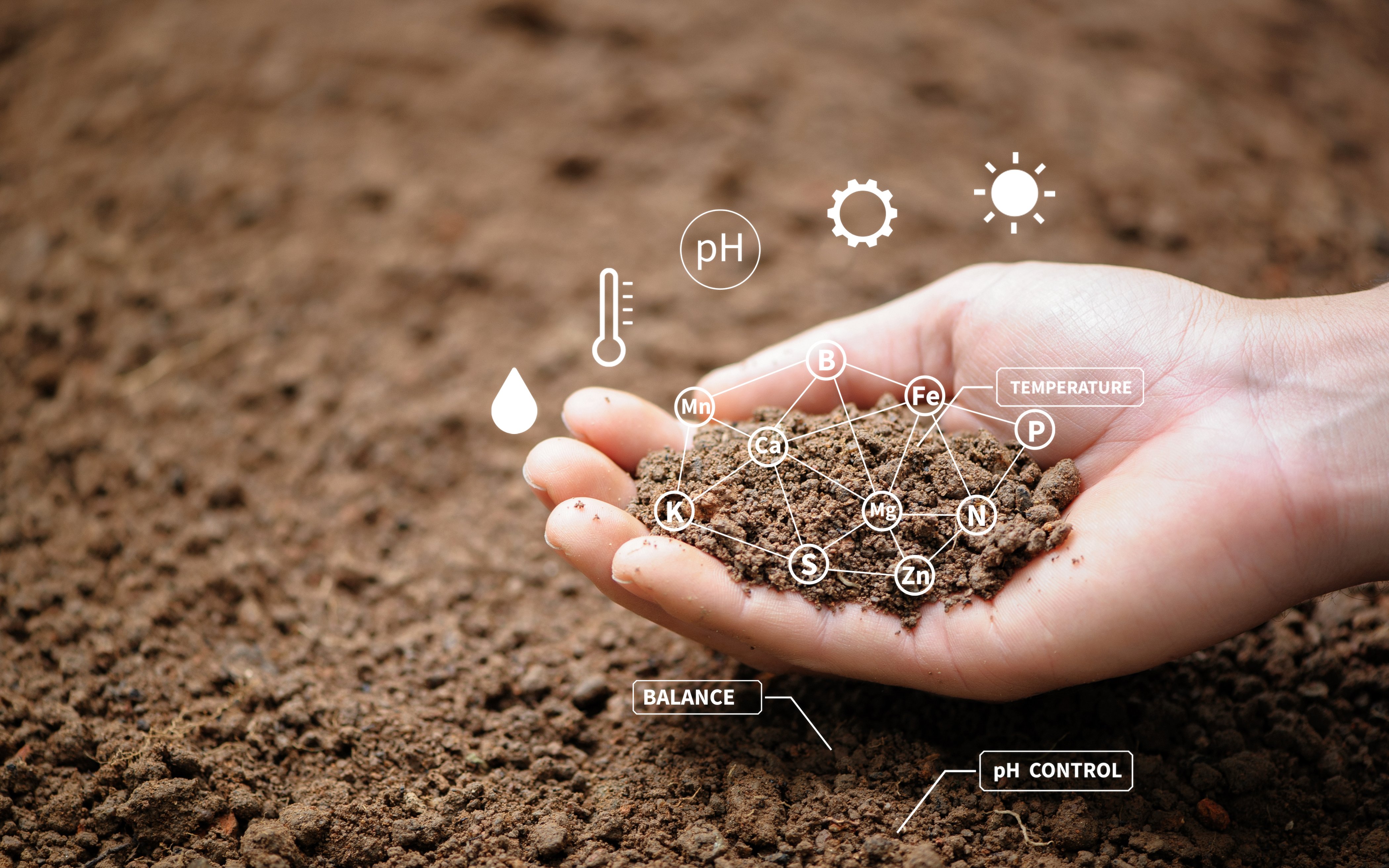
Sampling is unavoidable when we’re dealing with big data. The only way to find meaning from a large dataset is to make it smaller, and more manageable. However, data sampling can result in a biased or unrepresentative cross-section of data…which leads to inaccurate or misleading results. What we need is a way to generate a random, useful data sample that reveals powerful insight without making us slog through more data than we need to.
While there are algorithms for generating a random sample, they’re not always suitable to the dataset or the analysis that needs to be done. Moreover, on a massive dataset, they can take a lot of time. This leads to sampling shortcuts, and that leads to sample bias.
For example, if you listen to playlists on random you’ve probably noticed that one or two songs always seem to play more often than others. For music streaming, this isn’t a huge deal. But when we’re talking about a sample of data that you’re going to use to draw conclusions and build business strategies, it’s important to have the right sample.
So what does it really take to create an appropriate sample from your data? The answer to that question is pretty complicated.
There is no one perfect approach.
The right sample is dependent on your goals, so you can’t use the same process every time. Not very efficient, right?
Selecting an appropriate sample requires specific skills.
Data analysts don’t usually have the skills to generate data samples. Data scientists do have the skills to write code to find viable samples in big data, but is this their highest priority? Probably not. Because…
Producing a great sample takes time.
Writing sophisticated code and processing massive datasets takes time. And it’s probably time that your data scientists don’t have. So choices are made, and it’s likely that analysts are left working from unrepresentative samples.
Why is it so difficult to find a great sample in big data?
It's really hard to select a great, representative, unbiased sample because there are a lot of opportunities to make mistakes. But you can’t base your business strategy and future projects on half-baked data analysis.
A data scientist who sets out to build a great, representative sample knows there are tripping hazards just waiting in the wings:
Bias. Data sets may contain inherent biases that can affect the accuracy of the sample. For example, if a data set contains more data from certain regions, certain demographics, or certain time periods, a random sample may not accurately represent the entire population.
Dimensionality. Big data sets have a large number of variables, making it difficult to choose a representative subset for sampling. Choosing the wrong subset would lead to inaccurate conclusions.
Variability. Massive, complex data sets include a high degree of variability, making it difficult to draw conclusions from a small sample. For example, if the data set contains many outliers or extreme values, a small sample may not capture the full range of the data.
Overall, getting a good, useful sample in a massive, complex data set requires careful consideration of these factors, as well as an understanding of the underlying data and research questions. But the sheer effort will take a toll on your data scientists.
What if you could manage all of your big data?
Let’s walk away from the obstacles and consider what you could accomplish with a really great data sample. If you had a sample that you trusted to be random and representative, you’d be able to apply the right strategy to each problem or opportunity that you face. Your business leaders would be able to understand the real potential of new projects that are being considered. And your data science team would be preserved and prioritized for activities that matter the most.
Instead of burdening your data scientists with the task of creating the right data sample, we recommend using AI to find a truly useful data sample from your vast datasets…and to do it really fast. Virtualitics has built a collection of applications that will help you do just that, and we can’t wait to introduce it to you in the coming weeks. Follow us on LinkedIn so you don't miss the announcement! And if you're ready to learn more about Virtualitics just request a demo and we'll be in touch.