The AI Detective Continued: Using Intelligent Exploration to Identify True Crime Podcasts Worth Hearing
Written by Amanda Derrick
Feb 15, 2023 9:00:00 AM
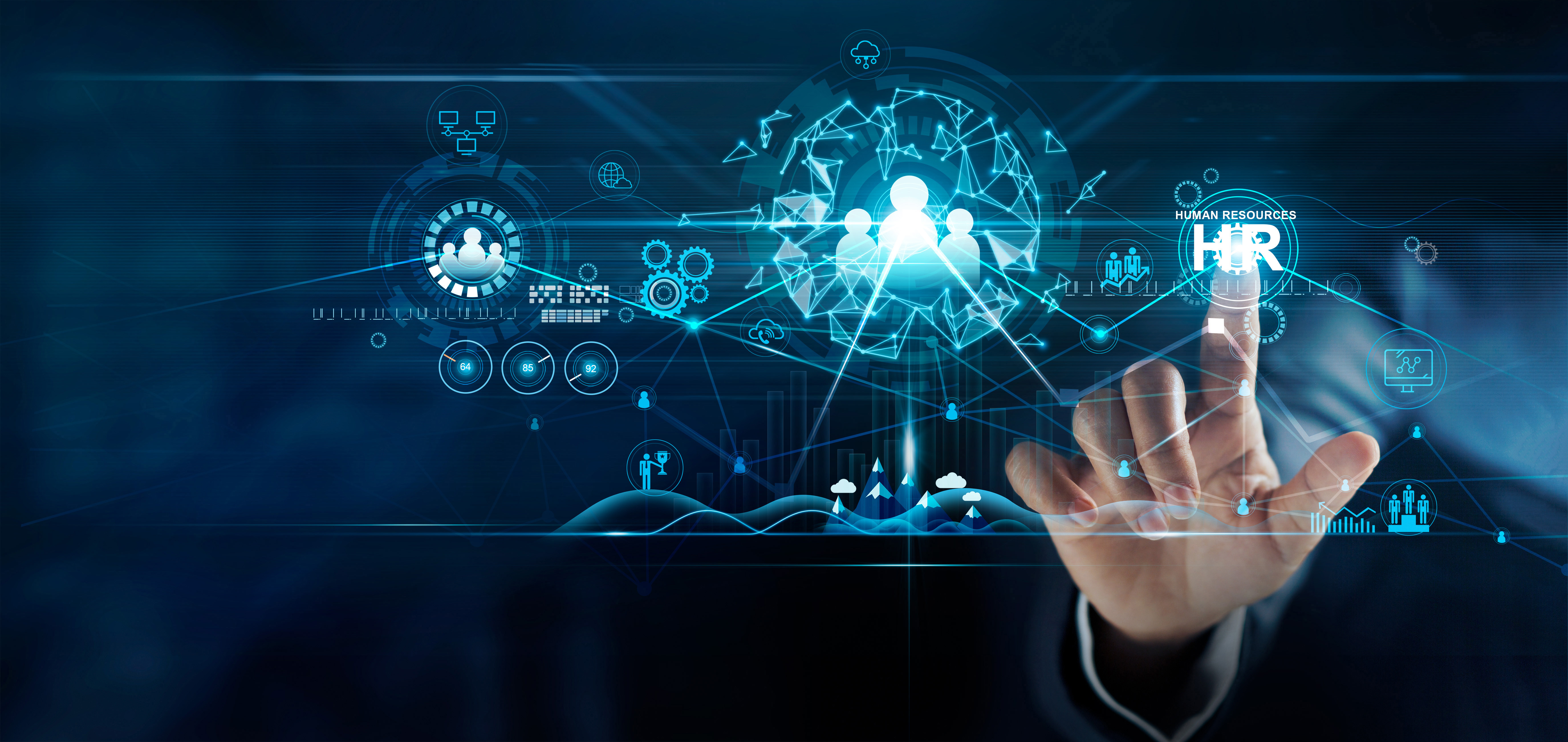
Artificial intelligence isn’t just about helping huge corporations with business strategies or discovering the next biomedical miracle. Machine learning is becoming part of everyday life, helping people save time, make smarter decisions, and remove their own biases to find answers they didn’t expect.
Take the example of identifying gripping, undiscovered true crime podcasts. Our first post on how AI can find the hidden gems in this popular genre was “elementary,” just scratching the surface of our technology and tools. Let’s dive deeper to see how Virtualitics’ Intelligent Exploration provides the power behind this fun analysis.
Don’t worry, this is for data nerds; no data science degree or criminal masterminding required.
Connecting dots between all relevant data
In our last “episode,” we started with a massive spreadsheet of data for over 2,000 true crime podcasts. Then, Max, our data science intern, used that data to create a Knowledge Graph on the Virtualitics AI Platform, illustrating communities and ties between different podcasts. Think of it as a giant, 3D crime board without the sticky notes or dry-erase markers. Oh, and you can spin it around and dive deeper to see more detail.
A knowledge graph combines a huge variety of data–including text–into one visual, showing groups of podcasts that share characteristics and relating them to other communities.
Max quickly saw that communities of true crime podcasts shared some interesting characteristics. And when he considered top performers, he saw some commonalities, like how top-ranked podcasts are almost always on Spotify and clock in at around 60 minutes.
But we didn’t just want to understand the most popular podcasts by analyzing numerical data. We wanted to dig into the meat of the subject. So Max leveraged built-in AI routines in Virtualitics—like Natural Language Processing—to investigate other podcasts that shared many of the same features as the most popular content.
How Natural Language Processing expands data analytics beyond math
To consider more than ratings, rankings, and categories for any product or service or content, you need a way to consider human language in your data. Human review and categorization of massive amounts of text take a lot of time and introduces bias. Natural Language Processing (NLP) is the application of linguistics, computer science, and AI to produce an output that we can analyze alongside numerical and categorical data. Being able to include text in our analysis with a simple click of a button allowed us to conduct a much richer, more meaningful investigation of the podcast data.
Using Virtualitics’ NLP application to parse the podcast descriptions, Max was able to highlight similar ones. He found that the most defining commonality was something unexpected: descriptions that included self-promotional content, like ACAST pages or links to the pods’ own websites.
No major themes in names. No common topics or content descriptions. So, what does this insight mean?
It means that there are no magic words that automatically tip us off to a great true crime podcast. But plenty of them take the time to create descriptions that can lead listeners to more content. They work to build an online community. This led Max to a next step in his legwork: investigating which podcasts had a social media presence using Smart Mapping.
Using Smart Mapping to show relationships between a lot of complex variables
Using a Virtualitics capability called Smart Mapping, Max found a direct correlation between Listen Score (like Nielsen ratings but for podcasts, included as one data source in our analysis) and podcasts that have Spotify, Twitter, and Google accounts. This tells us that for a podcast to get a high Listen Score (and be seen on top podcast lists) it must have an online presence beyond just publishing the podcast.
This view shows that a solid social presence is common to many of the top performing true crime podcasts.
Next, Max drilled down into the Spotify connection, to see what else we could learn:
What makes a great true crime podcast? This annotation illustrates the characteristics many of them have in common—a “Society + Culture” tag, Spotify presence, and explicit content.
This search showed us that Spotify seems to be the home of the highest-caliber true crime podcasts, based on Listen Score. Max then encoded the genres of podcasts (translation: making categorical names like mysteries, politics, and true crime readable by AI by mapping them to numbers) and ran our Smart Mapping feature again (don’t worry, all he had to do was click a button). He saw that “Society & Culture” is a prominent theme for podcasts with high Listen Scores, and that many of these pods are tagged “explicit” in their content.
Finally, Max looked at the relationship between length and the Google and Twitter presence of the podcasts:
There is pretty solid evidence that there IS an optimal length for a true crime podcast.
Regardless of how long a podcast has been around, top performers have episodes that sit right around 60 minutes in listening time. As true crime fans, we agree: Listeners don’t want short and sweet 15-minute episodes. We want to dive into the details on suspects, crime scenes, motives, and get enough information to entice us to keep listening.
Using Smart Mapping, Max was able to focus in on what makes a high-performing true crime podcast: published on Spotify, with ties to society and culture themes, not afraid to get their hands (or their words) a little messy, and ready to settle in for a longish discussion.
But this assessment is still relying on Listen Score as one of our key data points. What if there’s a great podcast that doesn’t fall in the top segment of options, that aren’t even assigned a Listen Score? What about that suspect hiding in plain sight?
Going beyond popularity: You are the company you keep
Guilt by association applies to both crime-solving and data analysis. We decided it was time to track down podcasts with high rankings in the vicinity (on the knowledge graph) of the most popular pods. If a series has many traits in common with those that are highly ranked, it’s reasonable to think it could be well worth a listen.
Max did this exploration using two different methods.
Method 1: Max started with podcasts that had high Listen Scores and related them to podcasts that had not been assigned a Listen Score (because they weren’t “popular” enough to earn one yet) using NLP similarity. This method showed us podcasts that have high engagement but are outliers in other ways. We like to call these eccentric (because they’re a bit out on their own), high-engagement podcasts. Here are a few this analysis revealed:
Method 2: Max considered podcasts that are in central communities, with a high similarity to other high performers, but don’t have a Listen Score. These could be hidden gems:
As in any good true crime story, there are caveats: A web presence doesn’t mean a podcast is good. Max discovered some strong performers with low Listen Scores, which seem to be driven by highly suspicious reviews.
Hmmm. Malfeasance discovered in our true crime research? It’s hard to think of anything more fitting.
You be Sherlock, we’ll be Watson
There are plenty of sites out there that rank and advertise true crime podcasts. But as lovers of the genre, we’re naturally suspicious. By using the Virtualitics AI Platform, Max led his own investigation with AI acting as his trusty assistant to organize information, prevent bias, spot anomalies, and present ideas for consideration and further exploration.
With great “right hand” technology, you can solve any mystery—including the ones hiding in data. In fact, we're going to solve the mystery of customer churn at an upcoming webinar. You can register to attend the webinar here, or go here to learn more about Intelligent Exploration and AI detective work.