The AI Detective: How AI-led data exploration helped us find our next binge-worthy podcast
Written by Amanda Derrick
Jan 24, 2023 9:00:00 AM
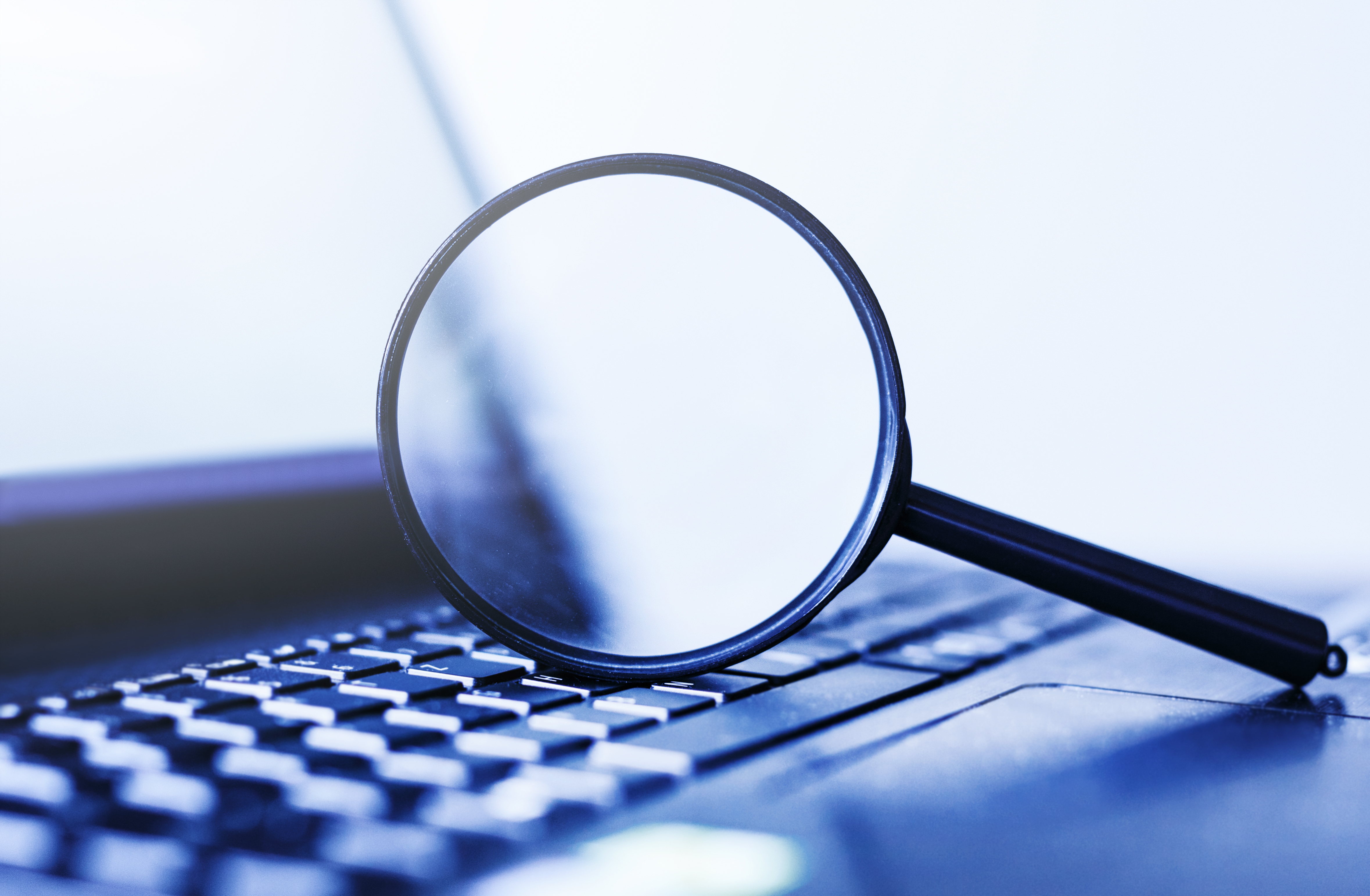
We love a good true crime story, and we’re always looking for something new. Could AI-led exploration help us sift through the thousands of podcasts out there and help us discover a hidden gem?
Let’s be honest, it’s pretty strange to ask where to find a “good” true crime story. Is there such a thing? We hope not. And yet, one of the most popular genres in podcasting is true crime. Our team is always swapping recommendations of what to listen to next. And the biggest podcasting platforms all provide rankings and charts for the most popular podcasts…including true crime. But we love being ahead of the curve and decided to do some sleuthing of our own, with the help of the Virtualitics AI Platform.
Collecting Leads
While sites like Spotify and Apple Podcasts don’t share how they determine which podcasts are at the top of their lists, they do tell us some of the data they use to determine their rankings:
- Follower counts—how many people subscribe?
- Number of unique listeners—how many people listen to your episodes?
- Completion rate—do people finish the episode once they start?
Do you know what they don’t consider? Podcast ratings, reviews, or the number of shared episodes. Essentially any kind of listener feedback other than subscription count is irrelevant, so making the top of the list really does boil down to a popularity contest.
We get it. Analyzing text and looking at those findings combined with other information is hard. But that doesn’t make the information irrelevant to the investigation. Being the curious data scientists that we are, we wanted to explore more data and see if we could learn more about what makes a really good true crime podcast—and which high performers might just be playing the numbers game.
We discovered Listen Notes, a website that collects a vast amount of podcast data. Additionally, it creates a Listen Score metric for top podcasts based on regular listening statistics combined with website activity, media mentions, reviews, and some other factors. We purchased the raw data, which included that unique metric as well as a ton of other information, and did some Intelligent Exploration of our own.
Ready to solve a mystery? Let’s dive in!
Zeroing in on the Data that Matters
Our search started out with a large spreadsheet that provided data for over 2,000 true crime podcasts. With 30+ attributes for each podcast, including the numerical rankings, categories, and text (the podcast description), it’s pretty tough to look at. Our Data Science Intern, Max, set out to make sense of this crime scene of a dataset.
Rather than rule anything out prematurely, Max used the Virtualitics AI Platform to create a Knowledge Graph that considered all the data we had; our own crime board!
A Knowledge Graph can combine different types of data into one visual, showing groups that have elements like runtime or streaming platforms in common.
What does this colorful cloud tell us? First, there is a lot of variety in true crime podcasts. But there are communities of podcasts that have characteristics in common, shown here by the clumps of data points. What kinds of interesting connections did we find in those communities?
- We learned that top-ranked podcasts are almost always on Spotify.
- Podcast listeners prefer their episodes to come in around 60 minutes.
- The name of a podcast doesn’t correlate to rating, so don’t judge a book by its cover.
- There are podcasts that share many of the same characteristics as top-performing ones but aren’t on the radar of the ranking charts. Likely suspects for a new favorite!
To unearth new finds that would feed our podcast addiction, Max leveraged the various built-in AI routines in Virtualitics to explore the podcasts and build his case for what makes the perfect crime podcast. Then he searched for other podcasts that shared those features but weren’t counted in the Listen Notes ranking score (they only rank a small portion of podcasts).
He discovered that many of the most popular podcasts shared characteristics with podcasts that weren’t making the “best of true crime” charts. We call these “eccentric high-engagement podcasts.” Eccentric is a geeky analytics term that means that these podcasts are out on the fringe of the network we created (that is, they’re not in one of those noticeable clumps of dots), but they are still characterized by high engagement amongst the listeners that they do have. Their fans love them, but they don’t yet rule the rankings. And it’s these podcasts that we see lots of potential for them to become the next big hit. Or at least our new obsession.
Using some really sophisticated (and just plain cool) investigative tools in the Virtualitics AI Platform, Max narrowed down our suspects from thousands to just six podcasts that our team can’t wait to try.
Want to know what we’re going to be listening to next?
- Manically Midwest. This one is focused on a specific geographical region, so if it’s a spot you’ve visited or have family in then it could be a really interesting listen.
- Twelfth House Tomfoollery. A great example of a high-rated niche topic, this podcast mixes in astrology as they chat about true crime, paranormal, and general mystery.
- Murder, She Cried. This one is unique because it has shorter episodes. If you’ve been wanting some true crime but don’t have 60 minutes to spare, this could be a great fit!
- Cup of Taboo. Topics from murder to cats! Fun and educational, with true crime mixed in.
- Father Knows Death. Max called this father/daughter duo “endearing” which is definitely a new term when it comes to true crime.
- Night Reader. With the tagline “We read in the dark” this podcast talks about scary topics from both true crime and fiction.
That’s the end of our investigation, but it certainly isn’t all the nitty-gritty details. We’ll share more of our analytics methods and processes soon! In the meantime, check out our own true crime fan and see how she’s finding more value in her data.