DATA VISUALIZATION, DATA ANALYSIS
Can Predictive Analysis Change Personalized Healthcare?
.png)
Written by Virtualitics
Dec 7, 2021 2:28:49 PM
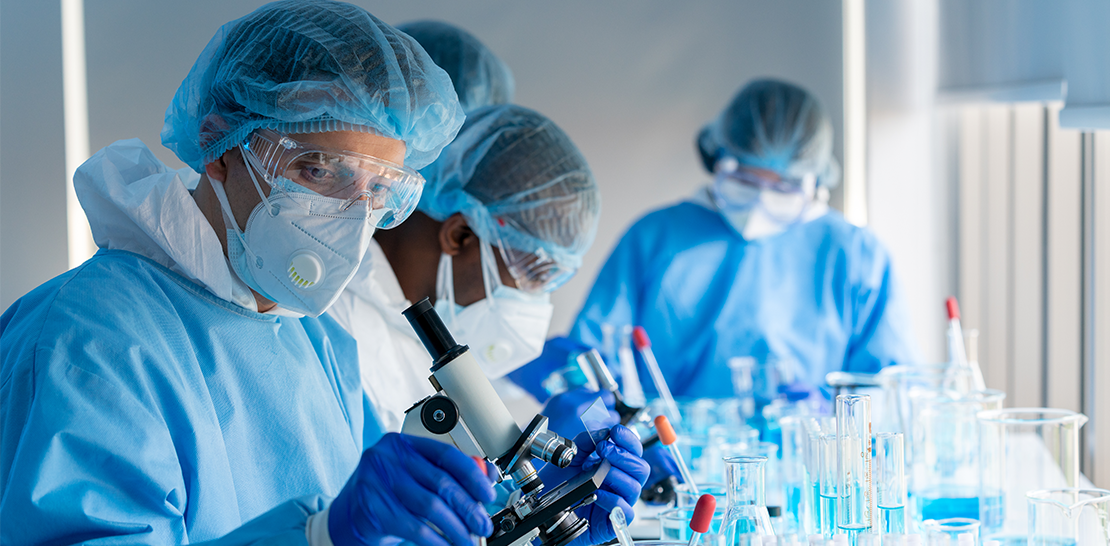
Data can be a powerful tool in any industry. It enables companies to understand the past and predict what might occur in the future. Predictive analytics attempts to answer the question, what will happen next? It uses modeling and forecasting to make those predictions with high probability. Its use in healthcare is wide-ranging, and one of the most important areas it can impact is personalization of healthcare for better outcomes.
The Role of Predictive Analytics in Healthcare
Predictive analytics leverages AI and machine learning to analyze historical data and identify patterns. Next, predictive algorithms and training models evaluate data across multiple sources. That data can derive from electronic health records (EHRs), administrative channels, health surveys, claims databases, medical alert services, patient registries, patient-generated from devices, and disease registries.
That’s a substantial amount of data, and it is continually growing. One patient can generate 80 megabytes of data each year. However, the volume is only one obstacle. Healthcare data lives in silos, and there are many issues regarding data sharing. Healthcare organizations need a platform to aggregate data and create data visualizations so that insights are actionable. Attaining this can reduce healthcare costs, improve patient care, and forecast outcomes (e.g., risk of disease or complications).
Predictive Analytics Is Revolutionizing Personalized Care
Every person is unique, regardless of whether or not they suffer from the same diseases or ailments. Diagnosis, prognosis, and treatment all look different depending on the specific patient. There are many standards around these components of care, but the more personalized they can be, the greater the likelihood of positive outcomes.
Here’s how each segment can benefit from predictive analytics.
Using Predictive Analytics in Healthcare Diagnosis
Predictive analytics can determine a patient’s likelihood of cancer or other diseases. If patients receive an early diagnosis, they can begin care faster, which improves their survival chances.
Using Predictive Analytics in Patient Prognosis
Clinicians can apply predictive analytics to determine if recurrence of disease or readmission after a hospital stay is likely. With this prediction, proactive intervention can occur.
All organizations want to prevent hospital readmission. It has a negative impact on many operational areas and patients. Predictive data analytics can combine data from various sources to identify those patients who might have complications when they return home. The data would look at patient risk factors, including social determinants of health (SDOH), to identify those with a high probability of complications. If that’s the case, providers can coordinate a care plan to prevent readmission.
An example is researchers using physiological data from the hospital stays of patients with congestive heart failure. They looked at data from 10,000 patients, determining that it could predict medically adverse events like readmissions.
Using Predictive Analytics to Determine Treatment Options
Not every patient will react the same way to treatment. The application of machine learning-based predictive analytic models can support treatment for patients with acute and chronic diseases.
In more immediacy, predictive analytics can provide insights to physicians at the time of care with real-time clinical decision-making support.
Regarding medication, precision medicine and genomics are becoming more critical. Predictive analytics modeling and simulating inform clinical trials to optimize dosing, predict product safety, and identify possible side effects. Biotech firm Isoplexis uses predictive analytics to connect the mechanisms and outcomes of therapies and disease.
More Ways Predictive Analytics Can Support Personalized Care
In addition to diagnosis, prognosis, and treatment, predictive analytics can also model hospital staffing needs and the availability of critical care beds in a proactive approach. It can also track hospital-acquired infection rates, which is critical in mitigating spread. Finally, it can also work to predict the maintenance of equipment to prevent failures.
Challenges of Predictive Analytics in Healthcare
Healthcare data is different from any other category. Regulations, including HIPAA, define the parameters around security and privacy. That can often be a barrier to greater data access.
Another primary challenge is the lack of expertise and technology. Healthcare analysts need advanced platforms to operationalize their processes. Without buy-in from leadership, they won’t receive the funding they need for people and technology.
Finally, healthcare data suffers from poor quality. There are no standardizations across EHRs or databases. The data may also be inaccurate and full of duplicates. Without proper data hygiene, it’s hard to get meaningful results.
Solving Some of the Challenges of Predictive Analytics for Better Patient Care
Our AI-driven data analytics and 3D visualization platform can’t resolve all the challenges of healthcare data. It can support the healthcare industry by making predictive analytics accessible that doesn’t require data scientists to uncover insights and make accurate predictions.