DATA ANALYSIS, DEPARTMENT OF DEFENSE, PREDICTIVE ANALYTICS
How to Bring in Past Data to Make Future Decisions
.png)
Written by Virtualitics
Jan 25, 2022 6:15:00 AM
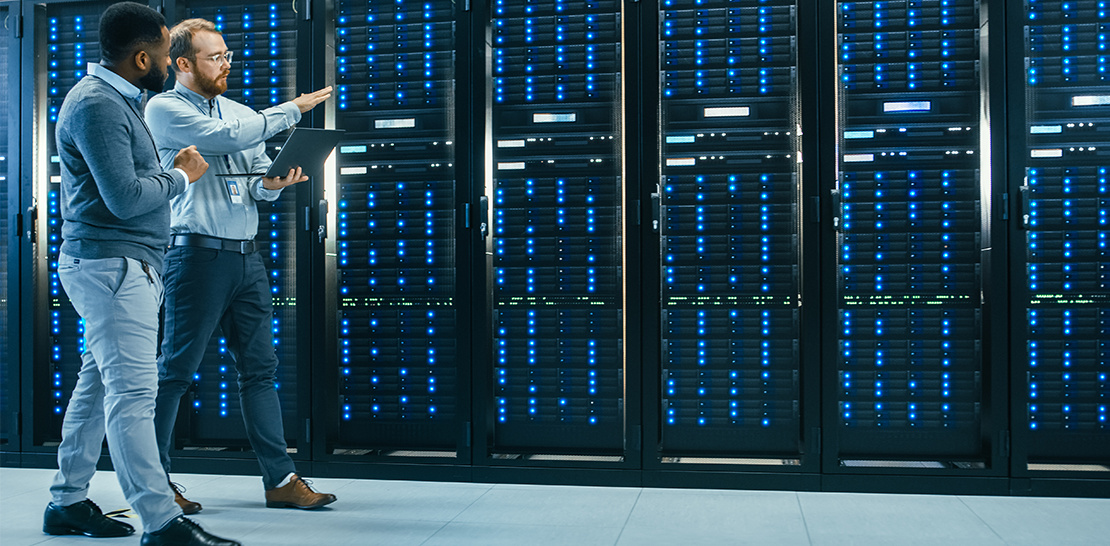
To achieve data-driven decision-making, you need all relevant data. The power behind prediction is the past. In any type of predictive AI modeling, you can’t derive insights and forecast the future of what will likely happen without including historical and current data.
But what is the easiest way to use this past data, and what considerations should you keep top of mind?
How Predictive Analytics Works and Why It Requires Past Data
Predictive analytics is an approach that organizations use to understand and identify patterns and trends from historical and current data. It leverages the data itself along with statistical modeling, machine learning, and AI.
From this analysis, you can detect both risks and opportunities, depending on the questions you’re asking. You may also find relationships between variables. For example, a popular use case for predictive analytics is consumer behavior patter analysis.
By including past and current data, companies can better predict what factors influence purchases and then personalize promotions to elicit the expected outcomes.
Without the knowledge of past consumer behavior data, it would be impossible to make such predictions. Garnering these insights is a smart tactic for businesses. A study by the Aberdeen Group as reported by Emerj found that companies that use predictive analytics were twice as likely to be able to target high-value customers and market their products to them exceptionally.
Although predictive analytics isn’t accurate 100 percent of the time, it is much more reliable than a hunch or an assumption. It paves the way for making critical decisions based on data. But how do you effectively group all your datasets?
How Does Data Ingestion Work?
To make sense of the data and make predictions, you have to feed the models data. That’s not always easy. Data can live in many places and be in various formats. The best way to approach such a task is to use a predictive modeling platform.
If your tool does these things, it will be relatively easy to move your historical data into a new environment where it can deliver actionable insights.
Predictive Analytics Platforms Allow You to Gather Data from Unexpected Sources
You may have third-party systems that hold lots of valuable past data, but exporting the data is not necessarily a perfect solution. In some cases, you may not have export access at all. What do you do then? In these situations, an integration will be necessary so that the two systems can exchange data.
If this is a top concern and has been holding you back from using predictive analytics, you’ll need to ask the right questions of both application owners. It is typically possible to integrate, and we’ve been able to do that for our users, including those who adopt our predictive maintenance solution.
Another concern may be integrating a data source that’s constantly changing, such as weather information. This kind of complex integration is critical in predictive maintenance because it can be a factor for assets in challenging environments. However, this concern shouldn’t limit you.
The bottom line is that most companies need to incorporate many data streams, and they won’t all behave in the same way. The key is to find a platform that can make this happen efficiently and effectively.
Discover the Power of Predictive Analytics with Diverse Data Sources
Predictive analytics can be a game changer for organizations. It can increase sales, reduce costs, mitigate risk, and much more.
To see the powerful results such a model can have, read our aviation company use case. The company used Virtualitics Predict to save $6-15 million in annual maintenance costs.