5 Ways You Might Be Introducing Bias Into Your Data... And What To Do About It
.png)
Written by Virtualitics
Nov 28, 2022 10:34:52 AM
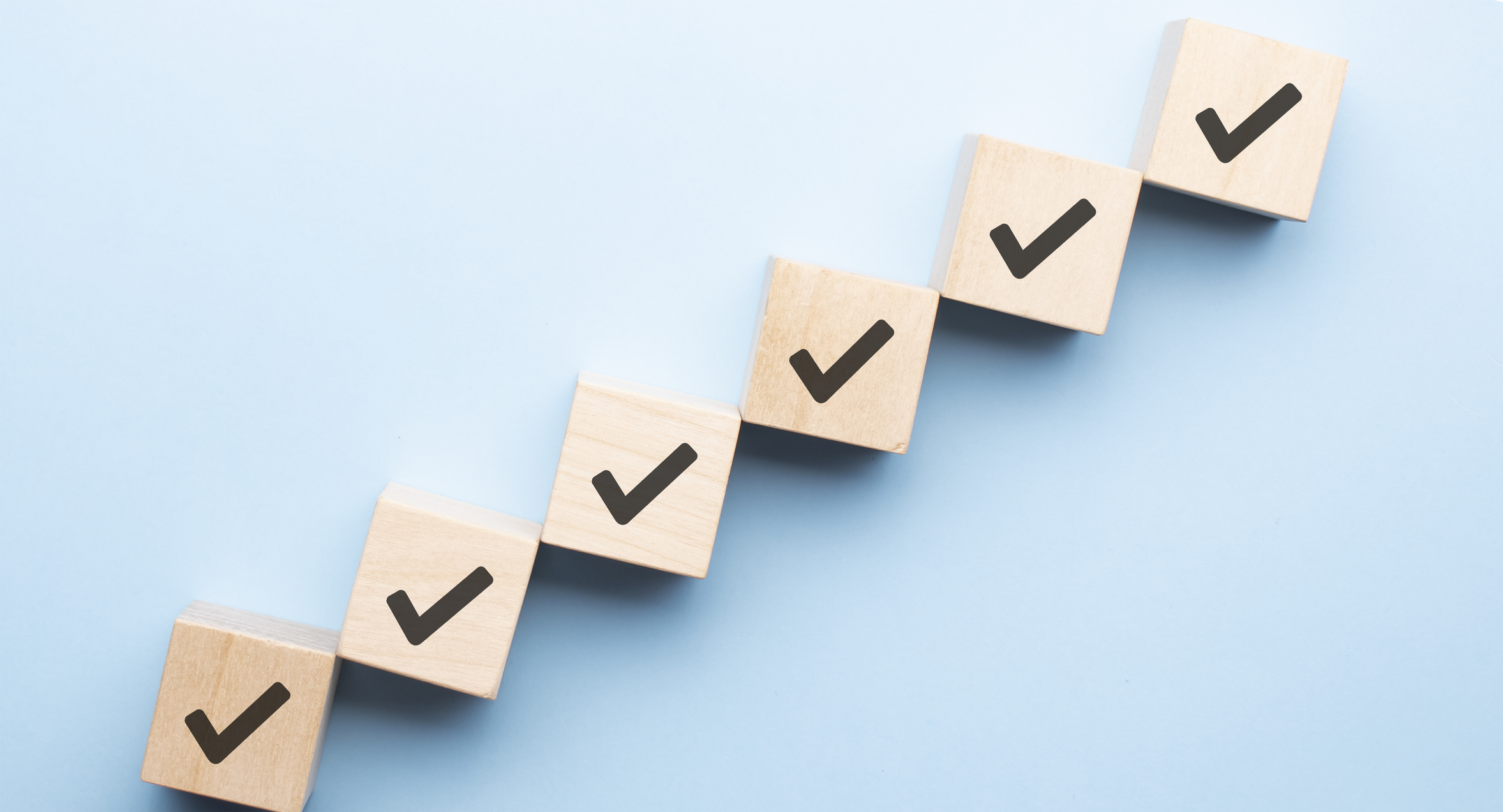
Much of the conversation around bias in AI has been on the bias that originates in the data and is then perpetuated by AI algorithms. For example, think of Amazon’s failed hiring algorithm that couldn’t overcome historic patterns of sexist hiring and found new ways to find–and exclude–women even when gender and names were omitted.
But racialized or gendered data aren’t the only source of bias.
Whether your goal is to create business-critical AI models or leverage your data to provide better analytics to help shape business strategy, bias is something you need to guard against proactively.
AI can actually be a tool in your arsenal to avoid bias if you use it from the start.
5 Common Sources of Bias in Data Analytics
We spent some time talking with data scientist and author, Tobias Zwingmann, about bias in analytics and he highlighted five common sources of bias.
1. Confirmation Bias
Confirmation bias is probably the most common source of bias and the easiest one to introduce. Most data projects kick off with data exploration based on a hypothesis. At best, the hypothesis-based exploration biases the exploration by limiting the scope of exploration, and the data that is explored.
For example, if you are exploring the impact of work-at-home policies on employee productivity, your exploration is going to focus just on data about employee work location, office schedule, and productivity metrics, neglecting other data sources that could impact results. You’ll paint a picture, albeit a very narrow one. But there’s also a risk that, in the absence of additional data, you’ll spot a correlation and draw the conclusion that supports your hypothesis at the expense of what’s really at play.
The productivity-monitoring software implemented by some organizations during COVID stay-at-home measures had the effect of demoralizing some employees to the point that their productivity was reduced. Suppose that data is left out of exploration because it didn’t fit the hypothesis. In that case, the analyst could draw the conclusion that working at home was the problem, rather than the heavy-handed monitoring system imposed on remote employees.
When your confirmation bias is really strong and you have a clear idea of what you want to find in your data, you’ll probably find it. In other words:
If you torture the data long enough, it will confess eventually.
“Most people are really not aware of …confirmation bias, which is so popular. But people just don't know that they have their assumptions and they don't want to explore data. They actually want to have their idea, or their hypothesis, confirmed. That's not data exploration.”
-Tobias Zwingmann
2. Survivorship Bias
In analytics, we tend to focus on the objects that survived or came through a process, and not those that were filtered out. This is a really easy trap to fall into because of course it’s easier to analyze the data that survives. We may feel like we don’t have enough detail of the objects that dropped out earlier in the process to include them. But it’s necessary to acknowledge and mitigate the fact that looking only at the group of survivors will give you limited insight.
For example, if you want to expand your sales and you focus your analyses on just your current customers, you will learn a lot about those who bought your product and why, but you won’t learn about those who didn’t. This will help you refine what’s currently working to continue to reach that same customer base, but if you need to reach a new audience you need to do something different. Only an analysis of those that didn’t survive can illustrate that.
3. Authority Bias
Authority bias is often seen in organizations with a strong hierarchy, but that is by no means the only place. This is the tendency for people to trust the opinion of a senior person and allow that opinion to influence our analysis so that we can produce insight that backs it up. It’s a version of confirmation bias–in this case, we’re motivated to confirm the bias of the authority figure.
4. Automation Bias
We trust automated systems more than non-automated ones and tend to treat the software or system as an authority. This bias can start to influence our analytics at the source if a downstream software solution is inputting values into your data. For example, grouping or classifying objects based on predefined criteria. By the time that data is being used in analytics, the analyst may not know where it came from or know enough to determine if it was applied properly.
5. Dunning-Kruger effect
Finally, we have the pernicious Dunning-Kruger effect that describes the phenomenon where people who know less in a certain area overestimate their knowledge and skill in that area. In fact, both sides of the Dunning-Kruger coin can result in biased analytics.
On the one hand, less experienced analysts who overestimate their abilities may look at a data set, see signals everywhere, and dismiss more likely explanations. And on the other, you have the seasoned analyst who’s looked at the data for years and when there is finally something of significance, they doubt whether it’s real.
How You Can Remove Bias
How can you remove or at least mitigate the bias in your analyses? Well, awareness of those biases is a strong first step. But practically speaking, consider making the following changes in your approach:
Set your hypothesis aside and broaden the scope of the data you explore to capture other possibilities.
Going back to our productivity example early on, any analysis of productivity and working from home should include data reflecting any other changes that occurred at that time such as software changes, policy changes, and staffing changes.
Create a data-first culture that supports analysts, even when they have to deliver results that contradict the opinion of an authority figure.
Way too much time and money are lost chasing up bad ideas. The analyst who can point out when something isn’t viable should be recognized for saving the organization. And if you do this in combination with the first point, casting a wider net, you may also be able to follow up with an even better option.
Augment your team with a solution that makes AI a co-pilot to act as a second set of eyes and insight.
In a platform like Virtualitics—which was purpose-built to support Intelligent Exploration—this goes beyond simply using out-of-the-box AI to do the analysis. AI is also used to apply the best visualization for the insight found and to surface statistically significant insights within the analyses, all with a click of a button.
You can learn more about how Intelligent Exploration casts a wider net here.
I see AI during explorations as something like my co-analyst. Something like a colleague that is like helping me to do this exploration… Maybe these are things which I have thought about… but maybe it's showing me something completely different. And then I suddenly realize...AI actually made some suggestions for me, that there might be some other things going on in the data which I wouldn't have thought about.
- Tobias Zwingmann