ROI is Everywhere: How Data Analysts Can Deliver More Value for Financial Business Leaders
Written by Amanda Derrick
Jun 29, 2023 9:06:27 AM
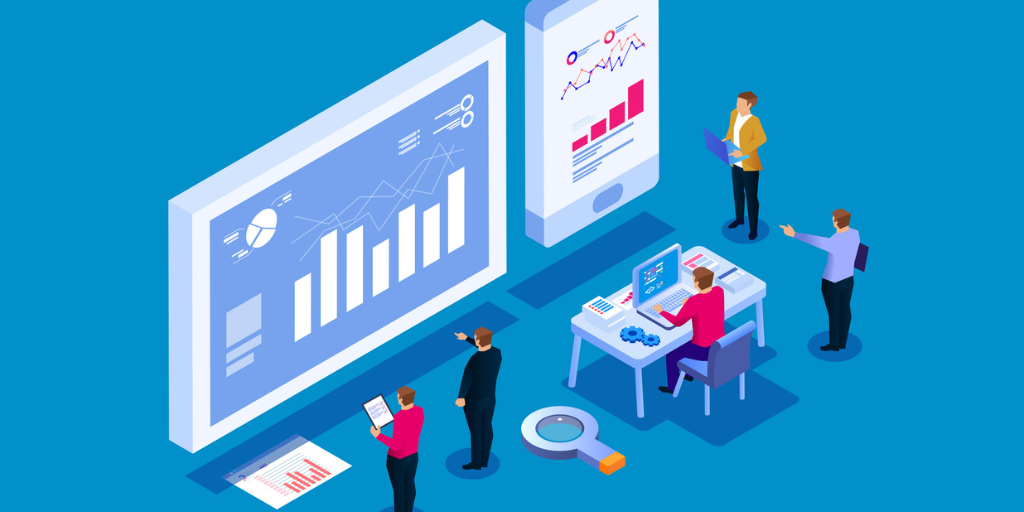
Data analysts can do so much more than just generate reports. Do your analysts have what it takes to be a major asset for financial services business leaders charged with crafting strategy? Here’s how analysts equipped with the latest AI-powered analytics tools are feeding decision-makers the insight they need, so they can make the right calls.
Strengthening collaboration and communication between data analysts and strategy leaders in financial services
AI-driven technology is opening huge doors in advanced data analytics. With AI to help manage the quantity and sources, all relevant data streams (proprietary, public, third-party) can be efficiently brought into a single analysis. AI also has the ability to transparently guide analysts to insights, putting more knowledge in their hands.
With that additional knowledge, analysts can help break down information silos that exist between departments, roles, and corporate divisions. Imagine a data platform that takes historical sales spreadsheets, order forecasts from business development, expense projections from accounting, sales rep CRM notes, and so on, synthesizes them in one place, and identifies points of interest and insight. With transparent insight and clear visuals, everyone starts seeing business problems holistically. This fosters organic, cross-functional partnerships among people who may not have had full visibility into one another’s information or pain points before.
There’s power—and profitability—in the ability to provide access to overarching insight by exploring multiple data sources. Let’s consider just a few ways that advanced data analytics tools can make analysts the partners that financial business leaders are looking for.
Advanced analytics tools can demystify customer segmentation
True customer analysis requires collaboration from data, business development, marketing, sales, information security, risk management, ESG, and legal teams.
The data streams to be evaluated might include third-party and public data like credit bureau information, customer reviews, government statistical databases, social media signals, and economic indicator forecasts. Then there is proprietary CRM data from surveys, purchase history, and sales conversations.
With AI-powered advanced analytics tools, all these data sources can be brought into a unified analysis. Users can see how many dimensions interact in easy-to-understand, 3D data visualizations, instead of comparing a few customer attributes at a time. Customer segmentation becomes far more multidimensional, granular, and beneficial when data analysts can organize prospects into segments and plot them in network graphs while investigating the relationships between data including:
- Demographics (age, gender, occupation, education level, marital status, and geographic location).
- Psychographics (customers' attitudes, beliefs, lifestyles, interests, and values, from surveys, interviews, and analysis of customer interactions and engagement).
- Financial data (income, spending habits, saving patterns, investment preferences, and credit history).
- Transactional data (purchase history, account balances, transaction frequency, and product usage patterns).
- Channel behavior (online banking, mobile apps, customer service calls, branch visits, engagement frequency, service inquiries, complaints).
- Risk preferences (investment goals, time horizons, past investment experiences, risk aversion levels).
- Past and upcoming life events (marriage, family births/deaths, retirement, home purchase, promotions).
With tools like the Virtualitics AI Platform, data analysts can intelligently explore what-ifs alongside those on the front lines of business strategy. AI automatically pulls forward what is statistically significant. Teams can use natural language queries to investigate further, change up their visualizations, and dive deep into their data to find the real causes behind customer churn.
Want to learn more about understanding customer behavior? Check out our webinar to dive into real customer churn examples.
Create a clear narrative to explain complex market analyses
Market analysis is another workflow that benefits from Intelligent Exploration of vast, interrelated financial services data sets.
Say a data analyst is tasked with evaluating the six-month outlook for market conditions and fluctuations to identify potential impact on investment portfolios. This could include assessing risks related to interest rates, commodity prices, exchange rates, and other variables like climate change disruptions that affect the value of assets or new investments. Coordination with finance, strategic planning, the head economist, risk managers, and others is necessary.
The data to support this analysis might include economic indicators, historical stock exchange behavior, tweets from influential economists, statements of regulators, government jobs reports, and more. Analysis can quickly go deep into the weeds, becoming difficult to explain to the non-expert. AI-guided data exploration shares analyses in logical narratives and intuitive visualizations automatically, so that everyone involved in decision-making can understand the insight present. The data analyst is able to get all contributors on the same page and leaders have the knowledge they need to set the direction for investment strategies.
Dig deep into data to understand insider threats
Managing insider threats takes coordination across many roles, from HR to risk management to infosec to compliance. It also requires analyzing a lot of big data sources to suss out patterns and correlations: employee behavior monitoring systems, audit trails, access controls, performance reviews, and more.
Data analysts can use AI-guided intelligent exploration to define different communities within the workforce, find commonalities among employees with anomalous behavior, and start to assess the difference between unintentional mistakes and true red flags.
Network extraction technology allows an analyst to create a 3D visual representation of the people and factors that contribute to insider risk. All kinds of dimensions can be graphed: unexpected login hours, large file transfers, length of employment, and hours of cybersecurity training completed.
This information can guide decision-makers on how to enhance risk management strategies, adapt internal controls, and improve operational efficiency. Maybe there’s so much friction in remote desktop access controls for hybrid workers that authentication protocols need an update. Maybe more training on data security is needed due to upticks in spear phishing by bad actors. Network graphs coupled with AI can visualize at-risk communities, then AI can be queried to offer recommendations on what to do next.
Give data analysts the tools to get more value from financial data
Sixty percent of data science and analytics leaders in a recent CIO.com survey say their organizations’ data is not being used to the fullest, and more than three-quarters of respondents are prioritizing upskilling analysts to do advanced analysis. AI-powered advanced analytics tools allow organizations to do just that.
Are you ready to improve information sharing between data analytics teams and business leaders? The ability to consider all the relevant data and present findings in ways that are clear, concise, and regulatory-compliant is within reach. Download our new e-book to learn how to unleash the power of your financial data analysts.