MACHINE LEARNING (ML), BIOTECHNOLOGY
Using Machine Learning in Healthcare
.png)
Written by Virtualitics
Nov 16, 2021 6:20:00 AM
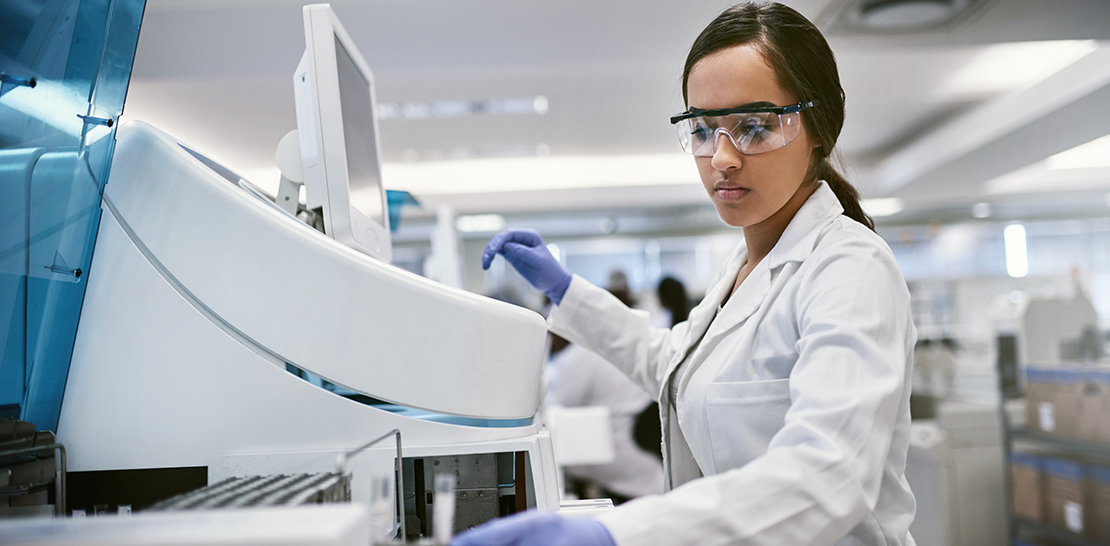
The healthcare industry generates massive amounts of data. Unlocking its value can, however, be challenging. Although the healthcare industry shifted to digitization years ago with electronic health records (EHRs), there are still many obstacles, including exchanging data and compliance regulations. Machine learning in healthcare expands and touches many areas, deriving powerful insights and predictions from data models.
With machine learning, the healthcare industry has the potential to improve operations, reduce costs, drive better patient care, understand public health holistically, and do much more.
Applying Machine Learning in Healthcare
So how does healthcare use machine learning? First, it’s important to define machine learning.
Machine learning is the use and development of computer systems that can learn and adapt by using algorithms and statistical models to analyze data patterns.
The process applies those algorithms to structured and unstructured data, uncovering interrelationships, anomalies, and trends. Algorithms learn over time as they ingest more data, imitating human learning and improving their accuracy.
This technology can provide many advantages to various fields of healthcare.
Precision Medicine
A common use of machine learning in healthcare is precision medicine, which describes the prediction of a treatment protocol's likelihood of success based on patient attributes.
One example is a study conducted on the treatment of acute myeloid leukemia. The researchers included data from patients with the disease to determine how and why pathologically similar cancers react differently to the same drug regimens. They also incorporated multiomic information. The study concluded with the assertion that this method performed better than other approaches.
What the researchers learned in this scenario allowed for future patients to receive more personalized care. With this use case, clinicians were likely able to save or prolong the lives of many patients.
Diagnosis
One of the most effective areas of machine learning in healthcare is diagnosis. Many cancers and genetic diseases are hard to detect, even with modern science. IBM Watson Genomics is an example of this, combining cognitive computing with genome-based tumor sequencing.
It’s also proficient at diagnosing diabetes, one of the most deadly and prevalent chronic diseases. IBM Watson Genomics can detect diabetes early so treatment can begin much sooner, resulting in better outcomes for patients.
Radiology Analysis
Another avenue for machine learning in healthcare is through radiology. There are several applications for machine learning in diagnostic imaging, including:
-
Optimized order scheduling and patient screening, which can reduce the risk of patients missing care
-
Intelligent imaging systems, leading to decreased image time and unnecessary imaging and also improving positioning
-
Automated detection and interpretation of findings for various cancers and other diseases with faster processing speeds and the ability to detect anomalies beyond what the human eye can see
-
Postprocessing, including image segmentation, registration, and quantification
-
Automated clinical decision support and examination protocoling
EHRs
EHRs are the standard houses for all medical records. Although this technology has many benefits, not all clinicians find it to be an efficient or effective tool. Now, machine learning is addressing these issues. For example, algorithms support clinician use by offering clinical decision support, image analysis automation, and telehealth technologies integration.
Medical Research
In situations where large amounts of health data are available, machine learning can also play a key role in determining trends in public health. Such data must be anonymized to ensure compliance. Crowdsourcing is one way of collecting medical data in order to analyze it. Findings from this could be significant, especially with the world still being in the midst of a pandemic.
Why Healthcare Entities Haven’t Fully Adopted Machine Learning
With all the possibilities that machine learning brings to the table, why isn’t every healthcare organization embracing it?
The key challenges are data governance, data silos, data scientist hiring and training, data integrity, compliance, and costs. Not every organization can launch machine learning initiatives. It seems out of reach for many, but new platforms are making it easier and more cost-effective.
Machine Learning in Healthcare Made Easier with 3D Visualizations
It is possible to overcome some of the biggest obstacles for healthcare to realize the power of machine learning. New platforms gather and clean the data, ingest it, and deliver visualizations. Data scientists aren’t necessary, which means it’s more accessible and actionable.