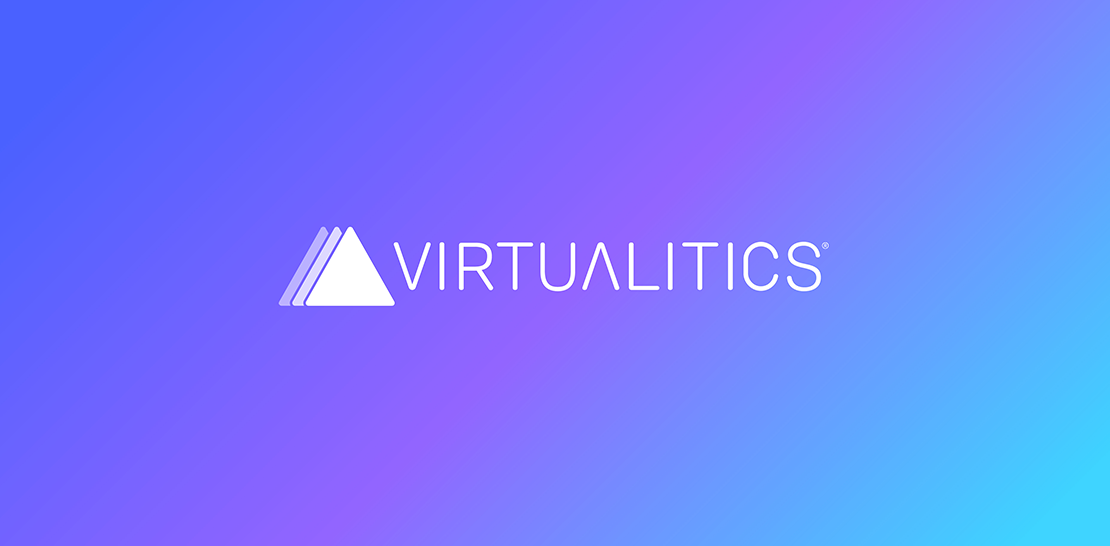
Data can be a valuable, powerful asset for organizations. However, its proliferation and complexities don’t always drive actionable insights. The more robust your data set is, the harder it is to analyze with traditional methods. Multidimensional data describes such a data set in a way that allows you to look at it beyond traditional two-dimensional structures.
It can help you uncover critical information that leads to better business decision-making.
Multidimensional Data Defined
Multidimensional data is a data set with many different columns, also called features or attributes. The more columns in the data set, the more likely you are to discover hidden insights. In this case, two-dimensional analysis falls flat.
Think of this data as being in a cube on multiple planes. It organizes the many attributes and enables users to dig deeper into probable trends or patterns. You can interrogate queries rather than just submit them, as practiced in relational databases. It’s a comparatively fast exercise, manipulating the different dimensions and perspectives by attribute.
What Are the Advantages of Multidimensional Data?
In simple terms, lots of dimensions deliver lots of information. As a result, the potential for insight increases. In working with this type of data model, you can realize many benefits, including the following:
- Group similar information: All like information combines into a single dimension. This process keeps things organized and makes it easy to view and compare.
- Visualize the output of complex data: When working with this model in a platform, you can visually recognize the outcomes of data analysis.
- Identify patterns, trends, outliers, and anomalies: There are no hidden data points in this “cubed” data visualization. You have everything grouped and organized, so you can more easily recognize the insights. You’ll be able to build interactive reports for these elements.
- Examine relationships among data from multiple sources: You can add many layers of source data to build your cube and discover patterns.
- Improve collaboration and analysis across geographic locations: By using this model, you can more easily work with users with tools in a shared virtual office. It's secure and allows people from different locations to work on the data at the same time.
- Extract valuable information from unstructured data: Unstructured data can be a pain point in analysis, but it’s no longer an issue with multidimensional data tools.
- Add “explainability” for non-data scientist stakeholders: Explaining data in an approachable manner is critical to driving better decision-making across the enterprise. Multidimensional data makes this possible.
- Compare the impact of changes to variables on the target data easily: If you want to understand the effect of change on specific data, you can with little strain.
- Process data quickly: You’ll gain speed with multidimensional data versus a relational database. The setup may be longer, but in the end, your processing will be faster.
- Maintain it with little work: Because you’re storing data in the same way it’s viewed, maintenance is simple.
- Save money: Multidimensional data models perform better and are more cost-effective than relational ones.
What Are Multidimensional Data Uses Cases?
When should your business leverage multidimensional data? Let’s look at a few use cases.
Improving Target Marketing
Every marketer wants to personalize communication and offerings to specific customers based on a host of data sets. Those may include demographics, consumer behavior trends, and detailed information about a customer’s past interactions with your brand.
With all these attributes, it can be challenging to see correlations. With multidimensional data models, you see the complete picture, which can lead you to conclusions. For example, you may find that time of day influences how big an order is, which may influence when you send out email offers.
Increasing Uptime with Predictive Maintenance
Predicting when equipment needs maintenance before it breaks or fails is key to minimizing downtime. Maintenance indicators vary widely depending on the complexity of the machinery. The data required to develop predictive maintenance strategies include maintenance logs, sensor data (if applicable), and other historical third-party data such as weather.
A great example of this is predictive maintenance for airplanes. These are sophisticated, expensive machines that have specific requirements for maintenance. In a case study on aviation, you can see how our technology used multiple data sources to build a multidimensional data model that helped predict what parts would require service.
Analyzing Complex Biological Data
Multiomics is another use case for multidimensional data. The data sets are all “omes”—genome, proteome, microbiome, and so on—related to health and disease analysis. As you can see, there are many features of the data, and it’s very complex. In traditional databases, there are limitations for researchers because they can only isolate single data points.
The key is the integration of data, which is difficult. This data model can support this process. Such a configuration allows researchers to understand public health better and discern correlations that fill current gaps in knowledge.
Experience the Power of Multidimensional Data
Watch the on-demand webinar “Machine Learning Model Explainability Using 3D Visualizations” to learn how machine learning-driven three-dimensional visualizations unleash the power of your data and drive more insights beyond two-dimensional views.
Ready to learn more?
Join us for a quick demo of our Intelligent Exploration capabilities and see how Virtualitics helps you explore your multidimensional data.